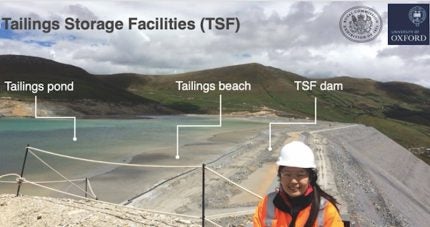
The World Bank estimates three billion tons of metals are required for the energy transition. This is a huge challenge set to the mining industry, especially as metals make up a tiny portion of the material that is dug out of the ground, with over 98% being waste, such as in copper mining. The mine waste, also known as tailings, is often stored behind huge dams called tailings dams or tailings storage facilities (TSFs). Because mining is one of the oldest industries, there are more than 30,000 TSFs in the world – a quarter of which are abandoned with nobody monitoring them. If these dams collapse, the results can be catastrophic.
Therefore, the often-forgotten consequence of our metal hungry future is the handling of the enormous amount of resulting mine waste. But what if any dangerous signals or changes to the health of tailings dams can be diagnosed straight away and any human error discovered immediately? What if decisions can finally be made in a way that consider long term consequences as well as alternative scenarios that help prepare for extreme events? This is the promise of Digital Twins: virtual replicas of the physical asset developed and updated using Machine Learning (ML) and sensor technologies both on the ground and in space.
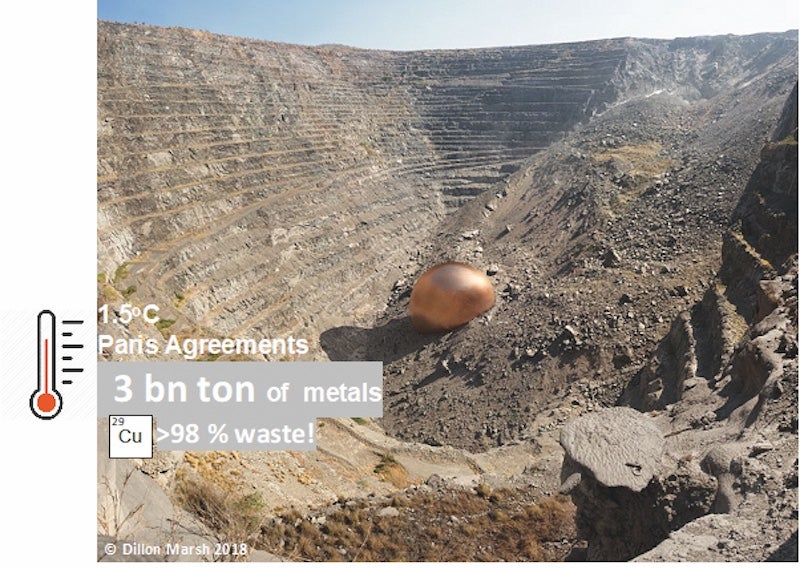
Our research ambitions are on developing a satellite based remote early warning system for these structures and which brings together three separate fields – geotechnical engineering, satellite remote sensing and machine learning within a digital twin system – and aims to push the limits of what is technologically possible. The research themes intend to address many of the fundamental questions that still need answering before a true digital twin system can be achieved for TSFs.
The latest satellite technologies offer many advantages to TSF monitoring and are complementary to pre-existing ground-based instrumentation and geotechnical modelling. Therefore, the initial function of a digital twin system lies in data fusion – combining many sources of data. In Bayaraa et al. [2022], we have created the first systematic demonstration of the complementarity of ground deformation monitoring for a failed TSF case study using Sentinel-1 InSAR (Interferometric Synthetic Aperture Radar), finite element modelling and ground-based prism data.
Although digital models such as building information models (BIMs) are common in mining, the key difference between a true digital twin and any other digital model is in its ability to update itself continuously to the conditions of the physical twin as the physical twin changes and vice versa. This puts an emphasis on the steps that do the checking to ensure that various stages of the planned construction and consolidation cycles have been completed (2]. Both optical and SAR satellite data offer a vantage view of these ’check phases’ with a rating on the degree to which the implementation is following designed intent. This ability to compare as-designed vs. as-implemented on the ground is crucial for identifying any human error that may occur and result in unexpected TSF behaviours.
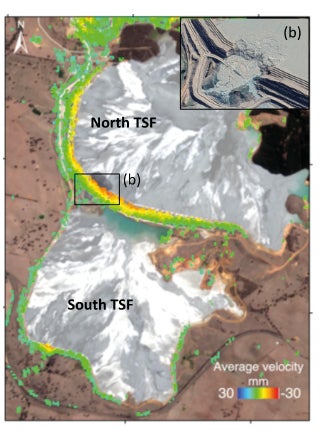
The real value of digital twins is in their ability to forecast and simulate alternative future scenarios, so that the best actions are recommended for decision making. The promise of truly data-driven decision making is the ability to understand the potential impacts of a decision in one part of the system within the context of the system as a whole. This is especially crucial for ensuring the safety of TSFs and their surrounding environment. For this to happen, the various physical conditions of the TSF needs to be continuously monitored, enabling the instant discovery of any faults and human error. The raw data collected from many different types of ground and remote sensors need to be fused, analysed, and interpreted into useful insight. Therefore, the enormity of this task may be feasible only with the promise of various ML tools at its core.
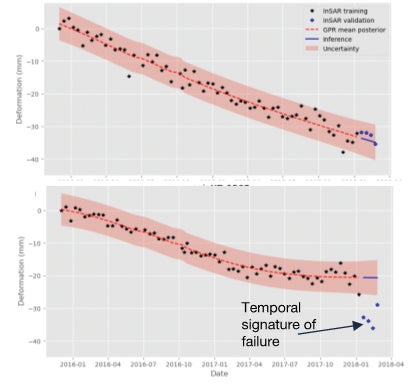
Critical role for tailings dams
On one end of the spectrum, government, community, NGOs, and investor stakeholders do not have access to TSF geotechnical data like the mining companies. However, the generation of a rigorous geotechnical model is resource intensive and contains uncertainties related to assumptions of the model parameters. Medium resolution Synthetic Aperture Radar (SAR) satellite data is available globally and open source from various space agencies. There are also commercial alternatives, which are often at higher resolution but are not globally available and are expensive to access. These advantages and limitations of the monitoring and modelling technologies are like a puzzle that requires research on how to fit them together.
Space and satellite technologies have a critical role to play in addressing some of the most pressing issues of today, here on earth, including addressing the climate emergency, ensuring food security and metal resources. I like to think of satellites and Earth Observation EO as ‘macro-scopes’ floating around the earth. Just like the way microscopes have given us insight into the world on the tiny, ‘micro’-scale and helped solve many diseases, EO macro-scopes are helping our understanding on the larger scale. Satellites were one of the first instruments that helped quantify and bring attention to the changing climate -which was recently recognised with Antarctic glaciers being named after satellites.
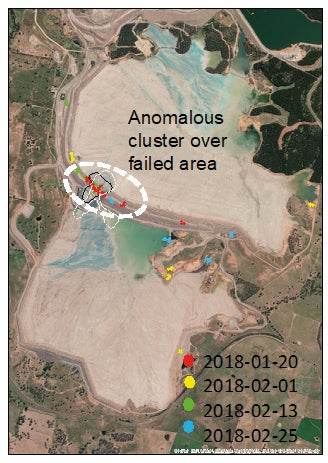
References
3) AI work on TSF early warning: https://www.mdpi.com/2072-4292/15/20/4910
Author information
Maral Bayaraa is a DPhil researcher at the University of Oxford and a senior Earth Observation Consultant at the Satellite Applications Catapult, the UK government’s innovation and technology hub. Her DPhil research has been awarded an Industrial Fellowship from the 1851 Royal Commission and an award at COP28 from the Prototypes for Humanity.
Maral says she is looking for research and industry collaboration:
• Collaboration and Data – A mine or a construction company – I would love to work with you. My research can help make the most out of your data and your data can help make sure our data and algorithms are working well. Create pilots and case studies together.
• Investment – My PhD research has only scratched the tip of the iceberg. So we need investments to make this global monitoring a reality.
• Communication – Poets and artists. We need help to communicate our science with current and future generations.
To get in touch, connect via her website:
https://maral.space/ or LinkedIn: https://www.linkedin.com/in/maralbayaraa/