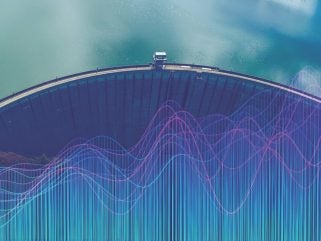
Climate change and extreme rainfall conditions are adding to the complexity of dam and downstream river operations, prompting greater interest in more scientific and intelligent data-driven watershed management. And within this current era of digital transformation, there is increasing curiosity about whether efficiency and productivity can be increased by digitalising traditional knowledge-based watershed and water infrastructure management.
DongSoon Park and Hojun You from the K-Water Research Institute in the Republic of Korea say that like many other sectors, the water management one is experiencing a wave of significant digital innovations. Although various endeavours have utilised cutting-edge digital technologies and explored more efficient and intelligent platforms for water resource management, the researchers claim to the best of their knowledge, there have been few attempts to apply digital twin technology across an entire dam and river basin, for comprehensive digitalised smart water resource management and data-driven decision-making.
What are digital twins?
A virtual digital model of an asset, process, or service in the real world, digital twin technology is described as being an interaction between the physical and digital worlds.
“This connection and communication between reality and digitalised features,” Park and You explain, “allows for real-time monitoring, intuitive understanding of situations, the possibility to identify any issue before it occurs, and various simulations and prediction modelling, facilitating optimal
decision-making.”
By enabling the simulation and testing of different scenarios in a virtual environment, the technology therefore reduces the need for costly physical testing and experimentation. It can also be a useful tool to improve the efficiency of the operation and maintenance of water infrastructure such as dams
and rivers.
In their research published in the journal Water, Park and You present an innovative digital twin dam and watershed management platform called K-Twin SJ. Designed in May 2021 and completed in December 2022, it was used by internal customers at Korea Water Resources Corporation from April 2023.
Utilising real-time data and simulation models to support decision-making for flood response and water resource management, the platform includes a GIS-based geospatial digital twin of the entire Sumjin Dam and river water system in Korea. Encompassing a watershed area of 4913km2, river length of 173km, and 91 water infrastructures, it has high-precision geospatial topography and facility information for the dams and river.
Synchronising real-time data such as rainfall, dam and river water levels, flow rate, and closed-circuit television, the platform incorporates three hydraulic and hydrological simulation models for efficient dam operation considering river conditions.
The goal of such data-based smart water management is to solve many tasks that have been routinely carried out in the past, more intuitively, reliably, and quickly with the help of the digital world. The authors add that the advantages digital twins and the direction it could pursue in smart water resource management can be summarised by the following:
- Visualisation: Visualise the three-dimensional representation of geospatial information and data analysis results for easy understanding.
- Intuition: Intuitively acquire information or knowledge with the help of digitisation without a complex reasoning process.
- Data synchronisation: Increase productivity by providing real-time synchronisation of related data.
- Extract value and knowledge from information: Provide information with engineering value to users and contribute to rapid and reliable decision-making.
- Sustainable use and practice: A usability-based platform that practitioners can continuously and conveniently use in their actual work.
The authors conclude that further research is required for future verification and validation of their platform, and this will take time.
Overcoming challenges
Managing a hydropower facility with so many components to oversee can be a cumbersome and complicated process.
“Hydropower facilities are like snowflakes; even individual turbines within a plant are unique due to their individualised construction and varying upgrades over the years,” says Nathan Fletcher, senior hydropower engineer at the Department of Energy’s Pacific Northwest National Laboratory in the US.
And this is where hydropower digital twins solutions can help with such operational challenges. It is also why, after hearing dam operators’ repeated frustrations, experts from multiple domains collaborated and developed a platform called Digital Twins for Hydropower Systems in 2023 to reduce outages and extend the lifespan of a dam. With new updates released in September 2024, dam operators can now use the dashboard to adjust factors that can potentially wear down a turbine’s efficiency, like unexpected electricity demand or extreme water level changes.
Dam operators can customise their digital twin to reflect the uniqueness of each facility; they just need to upload their facility’s data and the Digital Twins for Hydropower Systems dashboard handles the analysis.
“Each dam requires a unique maintenance strategy to improve efficiency, and the new digital twins platform can provide those solutions,” said Chitra Sivaraman, PNNL principal investigator of the project. “The platform is both extensible and scalable – capable of adapting to new facilities, data and models.”
Digital twin models also tackle another challenge. “The average age of the nation’s dams is around 60 years, meaning multiple generations of employees have worked on each turbine. And knowledge is inevitably lost as seasoned employees retire and new employees join the team,” says Scott Warnick, electrical and automation systems engineer at PNNL and the technical lead on the digital twins project. Hydropower digital twins can record and simulate all changes made to the dam over subsequent years – passing down knowledge and helping future generations make decisions.
“The digital twins solution enables hydropower operators to simulate different scenarios, such as low water flow or varying water levels, and predict future performance or maintenance needs,” Warnick adds.
To build a digital twin that accurately represents real life, the team used real-time data from a hydropower generation unit at Alder Dam on the Nisqually River in western Washington state, operated by Tacoma Public Utilities (TPU).
Leading the modelling development is Hong Wang, principal investigator of the project at DOE’s Oak Ridge National Laboratory. He and the team collected data, such as the river’s pressure as it enters the hydropower facility, how fast the turbines spin and how much power the dam generates over time.
In the original version of the digital twin, dam operators could only observe how normal or expected conditions affected the dam’s mechanical parts. Now in version 2.0, operators have more control. They can adjust water levels, flow rates and turbine speed that might change based on weather, droughts or energy demand. With the ability to simulate both normal conditions and higher or lower water flow, the dam operators can home in on potential problems before they arise.
The updated digital twins model addresses another emerging need. As the US moves towards a sustainable grid and embraces more wind and solar energy, hydropower systems must be adaptable and responsive to support a stable grid. The platform is capable of extending the lifespan of US dams while at the same time integrating additional sources of renewable energy to the grid. With a digital twin solution, operators can simulate and review real-world power demand fluctuations. If the model shows that the conditions are optimal to run the turbines, operators can feel confident about proceeding, which maximises revenue.
“The digital twins dashboard paves the way for the digitalisation of hydropower systems -providing a critical tool for operators to simulate and optimise grid operation for increased penetration of renewables, such as solar and wind,” Wang said.
With continual collaboration with TPU, the team gains insight into ways to improve the model. While helping TPU operate more efficiently, updates to the dashboard mean it can represent a wider diversity
of turbines.
“The PNNL and ORNL teams have the mathematical and practical skills needed to solve complex digital twin problems,” said Greg Kenyon, automation engineering manager at TPU.
The team is also working with Chelan County Public Utility in north-central Washington state to collect and analyse years of operation data records from the Rocky Reach Dam to develop a digital twin. Just like for Alder Dam, Chelan County’s hydropower digital twins will provide operators with the ability to review performance monitoring and analysis, perform predictive maintenance and optimise energy production – all at zero cost.
The digital twin will help minimise the risk to perform on the real operation, such as load rejection, over-speed test and vibration at the unit start or stop stage. The team anticipates future projects expanding on the application of digital twins, addressing broader concerns such as biological buildup like sludge in coolers, along with the challenge in making them more environmentally friendly which are now common worries for many utilities.
Looking to the future, the vision for hydropower systems is a data-driven one where data analytics and predictive maintenance algorithms drive asset management. It is one where there are no unplanned outages and lost revenue but rather outages determined by data-driven maintenance schedules and equipment replacements.
The project is funded by DOE’s Water Power Technologies Office.
Mining matters
With limited application of digital twin technology within the mining industry over the past few years, the concept has not yet been fully utilised in tailings dam safety management, maintenance, and cost-effectiveness. However, researchers from South Africa and Zimbabwe have been developing a conceptual framework for a smart tailings dam stability monitoring tool.
The framework relies upon digital twin simulation and machine-learning (ML) techniques, and comprises four main components: real-time data collection, digital twin modelling, ML-based early detection and prediction, and intelligence-driven decision-support.
Compared to traditional monitoring methods such as routine inspections and time-based maintenance, this approach offers the advantage of not requiring direct human input for the acquisition, transmission and processing of data. As a result, much larger datasets can be manipulated and processed efficiently without any human error. Furthermore, the proposed method does not suffer the limitation of requiring physical presence at a mining site in order for data and output information to be accessed. Since the model is virtual, many of its operations can be carried out or accessed remotely, including data transmission, processing and trend visualisation.
Finally, the proposed approach allows for the cost-effective simulation of various parameter combinations to determine their collective impact on the stability of tailings storage facilities and potential for failure. There are several potential improvements and extensions that can be made to the proposed framework to enhance its performance and efficiency.
As the authors Mwanza et al suggest, one improvement could involve incorporating forecasts of weather elements, such as rainfall and wind speed, as well as seismic events, into the prediction model in order to improve its accuracy. Other improvements could be to incorporate site metadata, such as age of facility, dam dimensions, and material of construction into the model, as well as adoption of deep learning algorithms. This would likely improve the accuracy and reliability of the model even further.
The authors believe their proposed framework is an important contribution in current efforts to mitigate against tailings dam failures in the mining sector. It has the potential to transform the way in which tailings dam stability is monitored by allowing for more accurate and real-time monitoring of structures and ground conditions. Adoption of the model could lead to safer and more efficient mining operations, as well as better protection of environment and mining communities. Compared to traditional monitoring methods, it offers the means for developing a more accurate and comprehensive model of the tailings dam, and can provide early warning detection for the prevention of catastrophic failures.
Future research should focus on addressing the framework’s limitations and on developing strategies to make the framework more cost-effective and scalable for widespread adoption in the mining industry.