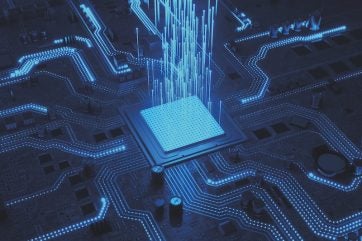
1. Internet of Things (IoT) sensors: the backbone of condition monitoring
At the heart of any condition monitoring system lies the network of sensors that continuously collect data from various components of the hydropower plant. These sensors – often IoT-enabled – track critical parameters such as vibration, temperature, pressure, water flow, and turbine speed in real-time.
- Vibration sensors: Vibration sensors are among the most widely used technologies in condition monitoring. Installed on turbines, generators, and bearings, these sensors detect deviations from normal operating conditions, indicating potential issues like misalignment, imbalance, or wear. Small changes in vibration patterns can be early signs of larger mechanical problems.
- Temperature sensors: Excessive heat is often a warning signal of malfunctioning equipment. Temperature sensors in critical components like turbines, transformers, and bearings monitor heat levels, alerting operators to rising temperatures before they can cause permanent damage.
- Water flow and pressure sensors: Hydropower generation depends heavily on consistent water flow and pressure levels. IoT sensors track these variables, ensuring optimal efficiency and safeguarding against sudden changes that could damage equipment.
The integration of IoT sensors enables hydropower plants to collect vast amounts of real-time data, providing actionable insights into equipment health. By deploying IoT technologies, operators gain constant visibility into plant conditions, allowing for timely interventions and reducing the need for manual inspections.
2. Predictive analytics: anticipating failures before they happen
With IoT sensors generating huge volumes of data, the next challenge lies in interpreting that information. This is where predictive analytics powered by artificial intelligence (AI) and machine learning (ML) comes into play.
Predictive analytics systems analyze historical and real-time data from the plant to detect trends and patterns that could indicate potential failures. These systems continuously learn from the data, improving their predictions over time.
- Failure prediction: One of the most significant advantages of predictive analytics is its ability to forecast equipment failures well in advance. For instance, by analyzing vibration data from a turbine over several years, an AI-driven system can predict when that turbine is likely to experience a breakdown, allowing operators to schedule maintenance before any serious damage occurs.
- Optimized maintenance schedules: Predictive analytics also allows hydropower plants to move away from traditional time-based maintenance schedules and adopt a more efficient, condition-based maintenance strategy. This not only saves costs but also prevents unnecessary downtime, ensuring that maintenance is performed only when truly needed.
Incorporating AI and machine learning into condition monitoring creates smarter, more proactive maintenance regimes that can anticipate issues before they disrupt operations, enhancing plant reliability and efficiency.
3. Acoustic emission monitoring: listening for early signs of damage
Another powerful technology increasingly being utilized in condition monitoring for hydropower is acoustic emission monitoring. This technology captures high-frequency sounds emitted by mechanical equipment when materials begin to degrade or cracks start to propagate.
- Structural health monitoring: In hydropower plants, acoustic sensors can be placed on turbines, generators, and even dam structures to detect early signs of material stress or fatigue. For example, if a turbine blade begins to develop microscopic cracks, it will emit high-frequency sound waves that are imperceptible to the human ear but detectable by acoustic sensors.
- Non-intrusive monitoring: One of the key benefits of acoustic emission monitoring is that it is non-intrusive. Unlike some other monitoring methods that may require disassembly or direct access to components, acoustic emission systems can detect issues from the surface, making it possible to identify problems without disrupting operations.
This technology provides an additional layer of protection for hydropower plants, allowing operators to catch early-stage issues that might otherwise go unnoticed until they become major failures.
4. Cloud computing and edge processing: managing the data deluge
The large amount of data generated by IoT sensors presents both an opportunity and a challenge for hydropower operators. Cloud computing and edge processing technologies help manage this data more efficiently, ensuring that critical information is available in real-time while reducing the burden on local infrastructure.
- Cloud-based analytics: Cloud platforms enable hydropower operators to store and process vast amounts of condition monitoring data securely. These platforms can run advanced analytics and machine learning models, providing real-time insights into the health of equipment and infrastructure from anywhere in the world. Operators can remotely access dashboards, receive alerts, and even monitor multiple plants simultaneously from a centralized system.
- Edge processing: While cloud computing offers great scalability, edge processing is essential for real-time decision-making. By processing data locally—closer to where it’s generated—edge devices can filter out noise, detect anomalies, and send only critical information to the cloud. This reduces latency, allowing for faster responses to urgent issues.
The combination of cloud and edge technologies ensures that hydropower plants can handle the growing volume of condition monitoring data while maintaining the speed and agility needed for critical operations.
5. Digital twins: simulating plant operations for enhanced monitoring
A cutting-edge innovation in the condition monitoring space is the development of digital twins – virtual replicas of physical systems. By creating a digital twin of a hydropower plant, operators can simulate plant operations, predict performance, and test different scenarios without affecting the real-world system.
- Real-Time simulation: A digital twin continuously receives data from IoT sensors, allowing it to simulate the exact conditions of the plant in real-time. If the twin detects any deviations from normal behavior, it can immediately alert operators, providing insights into the root cause of the issue.
- Scenario testing: Digital twins also enable operators to run “what-if” scenarios, testing the impact of different operating conditions or maintenance strategies. For example, operators can simulate how a reduction in water flow would affect turbine performance or predict how a delayed maintenance event might increase the likelihood of failure.
Digital twins represent the next frontier of condition monitoring, offering unprecedented levels of insight into plant operations and enabling more informed decision-making.
Conclusion
The technologies driving condition monitoring in hydropower – IoT sensors, predictive analytics, acoustic monitoring, cloud computing, edge processing, and digital twins – are revolutionizing how hydropower plants are managed. By integrating these advanced tools, plant operators can proactively identify issues, optimize maintenance schedules, and ensure long-term operational efficiency.