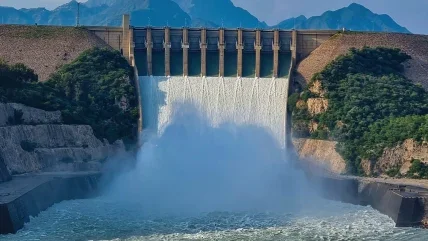
The definition of digital twin in Wikipedia says “A digital twin is a virtual representation that serves as the real-time digital counterpart of a physical object or process.” Indeed, the digital twin (DT) concept was initially introduced at the start of the 21st century with intent to create a digital model to reflect physical systems and derive insight from the model to make the decision on system operation. DT is also a promising enabling technology for realising smart manufacturing and industry 4.0. In general, DTs consist of three main parts: physical product, virtual product, and connected data that link physical and virtual product via various data communication schemes.
In recent years, industry organisations in multiple sectors have developed, tested, and utilised digital twins in their operations. Industry researchers expect the DT market to grow from US$3.8billion in 2019 to US$35.8billion by 2025, according to the latest report from https://www.marketsandmarkets.com/Market-Reports/digital-twin-market-225269522.html.
Today, DTs have been used in several areas, including design, production, prognostics and health management (PHM), etc.
- DTs in product design: DTs can be used to design new products in a more responsive, efficient, and informed manner and can also synchronise design and production through DTs. Researchers have developed DT models to manage geometrical variations, evaluate the quality of products at the early stage, and design production lines, etc. In product design, the traditional DT approach uses advanced physics-based simulation and optimisation, such as a CAD model for the first step. This is followed by simulation for the next step, including fluid dynamics simulation, finite element analysis, thermal dynamics, structural analysis, and optimization. Currently, the research and development of DTs also relies on machine learning and data-driven models, which take the information from machine sensor data or traditional digital twins. DTs based on data predict failures more quickly in different areas and also help engineers identify the sensitivity of their design, allowing them to generate new designs from existing digital twins.
- DTs in process operation: DT modeling can be used to monitor, control, and optimise production processes and make these processes more reliable and flexible.
- DTs in prognosis and health management (PHM): Currently, most of the research in DT applications is related to PHM. The first application of DTs was in PHM of aircraft, which was used to manage aircraft service life. The idea was to create a DT model to monitor the operational state of aircraft wings. In addition to the aircraft, the application of DTs in PHM is also extend to other fields, such as additive manufacturing processes to predict the cooling rate, velocity distribution, etc. Compared with traditional PHM, it has been observed that the DT-driven PHM is more accurate in predicting system faults and thus enables responsive and economic system maintenance.
Hydropower system challenges
With an average machine age of 64 years, the US hydropower fleet requires smart modernisation to reduce costs and enhance the overall reliability and value of the nation’s longest-serving renewable energy technology. Hydropower operations are becoming more complex and demanding as hydropower strives to provide grid reliability and resiliency in the face of variable renewable energy production provided by expanding solar and wind installations. As the electric power grid prioritises reliability, resiliency, and value amidst an evolving mix of variable renewable and baseload assets, hydropower technology will require the integration and full benefit of the best available and future advancements in sensors, data and control systems, analytics, simulation, optimisation, and computing capabilities to remain competitive. We refer to this need as the Hydropower Digitalisation Challenge.
Project overview
To address this challenge, the development of digital twins for hydropower systems is necessary. Funded by the Water Power Technologies Office of the US Department of Energy, Oak Ridge National Laboratory (ORNL) and Pacific Northwest National Laboratory (PNNL) launched a project in 2020 to develop a Digital Twin for Hydropower Systems (DTHS) open-platform framework (OPF) as a key initiating research activity and outcome.
The overall structure of the DTHS-OPF is shown in Figure 1, which shows that DTHS-OPF will collect data from real hydropower systems and continuously update its dynamic models for various components of hydropower systems. This enables the DT to comprehensively represent the actual plant operation in a digital form for its use in the above-mentioned three aspects. It also has a powerful user interface including visualisation, augmented reality, etc. to allow user-friendly functionalities for the hydropower industry, hydropower system equipment manufacturers, and academia.
Once completed, DT will perform in parallel operation on actual hydropower systems as it expands from a single generation unit to a fleet, and any operational dynamics and status will therefore be reflected and mimicked by DT. Therefore, operators in hydropower plant in the US hydropower industry, hydropower system equipment manufacturers, and academic institutions can use DTHS to:
- Optimise the actual plant operation in response to changes and requirements of the overall power grid for managing electrical power flows and demand.
- Initiate testing on operational optimisation before fully implementing these operational strategies.
- Using the real-time data from the plant, DT can serve as a basis for performing fault diagnosis, condition and health monitoring, and management of hydropower systems operation. This would allow economic and in-time repair of relevant equipment used in hydropower generation.
- Finally, as the models used in DTHS are constantly updated via the use of real-time data, DT will present a true reflection of system dynamics. This will enable other relevant research communities to use DTs as a platform to further the development of hydropower systems in terms of equipment design, control, and optimisation. For example, new algorithms on modelling and control can be developed using models and interface capabilities of DTHS-OPF.
Therefore, DTHS-OPF can be effectively used to modernise the hydropower industry as it moves toward full-scale digitalisation. It will help the US hydropower industry optimise its operation as an integrated and important part in the country’s power grid. It will have promising potential for predictive and prescriptive applications in the future.
ORNL and PNNL’s accomplishments, capabilities, and expertise make the joint team an effective choice for this effort. Indeed, ORNL and PNNL envision a future state, more than five years hence, in which the hydropower digitalization challenge is being met by a widely adopted, extensible digital twin for hydropower systems (DTHS) core product. It would be used as a best practice and baseline digitalisation technology for designing, operating, and managing the reliability, performance, and costs of most hydropower assets. The timeline of the project is shown in Figure 2 together with a website link at https://www.ornl.gov/content/digital-twin-hydropower-systems-project, where the team is seeking feedback and comments from industries and academia communities. ●
This manuscript has been co-authored by UT-Battelle, LLC, under contract DE-AC05-00OR22725 with the US Department of Energy (DOE). The US government retains and the publisher, by accepting the article for publication, acknowledges that the US government retains a nonexclusive, paid-up, irrevocable, worldwide license to publish or reproduce the published form of this manuscript, or allow others to do so, for US government purposes. DOE will provide public access to these results of federally sponsored research in accordance with the DOE Public Access Plan (http://energy.gov/downloads/doe-public-access-plan).